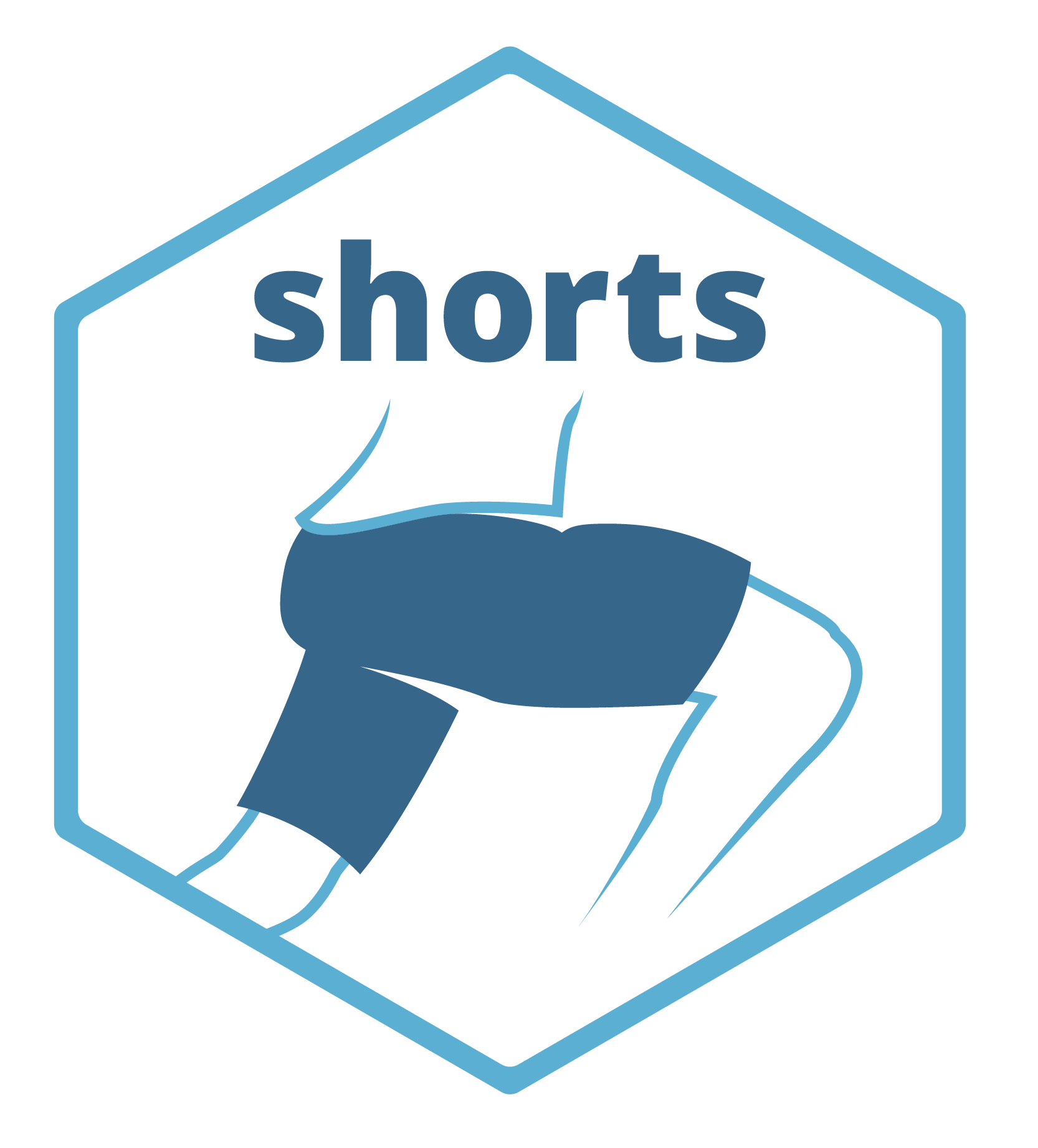
Model functions
Source:R/model-function.R
, R/model-in-situ.R
, R/model-radar-gun.R
, and 3 more
model_functions.Rd
Family of functions that serve a purpose of estimating short sprint parameters
model_in_situ
estimates short sprint parameters using velocity-acceleration trace,
provided by the monitoring systems like GPS or LPS. See references for the information
model_radar_gun
estimates short sprint parameters using time-velocity trace,
with additional parameter TC
serving as intercept
model_laser_gun
alias for model_radar_gun
model_tether
estimates short sprint parameters using distance-velocity trace
(e.g., tether devices).
model_tether_DC
estimates short sprint parameters using distance-velocity trace
(e.g., tether devices) with additional distance correction DC
parameter
model_time_distance
estimates short sprint parameters using time distance trace
model_time_distance_FD
estimates short sprint parameters using time-distance trace
with additional estimated flying distance correction parameter FD
model_time_distance_FD_fixed
estimates short sprint parameters using time-distance trace
with additional flying distance correction parameter FD
which
is fixed by the user
model_time_distance
estimates short sprint parameters using time distance trace
with additional time correction parameter TC
model_time_distance
estimates short sprint parameters using time distance trace
with additional distance correction parameter DC
model_time_distance
estimates short sprint parameters using time distance trace
with additional time correction TC
and distance correction TC
parameters
model_timing_gates
estimates short sprint parameters using distance-time trace
(e.g., timing gates/photo cells)
model_timing_gates_TC
estimates short sprint parameters using distance-time trace
(e.g., timing gates/photo cells), with additional time correction parameter TC
model_timing_gates_FD
estimates short sprint parameters using distance-time trace
(e.g., timing gates/photo cells), with additional estimated flying distance correction
parameter FD
model_timing_gates_FD_fixed
estimates short sprint parameters using distance-time trace
(e.g., timing gates/photo cells), with additional flying distance correction parameter FD
which
is fixed by the user
model_timing_gates_DC
estimates short sprint parameters using distance-time trace
(e.g., timing gates/photo cells), with additional distance correction parameter DC
model_timing_gates_TC_DC
estimates short sprint parameters using distance-time trace
(e.g., timing gates/photo cells), with additional time correction TC
and
distance correction DC
parameters
Usage
model_in_situ(
velocity,
acceleration,
weights = 1,
acceleration_threshold = 0,
velocity_threshold = NULL,
velocity_step = 0.2,
n_observations = 2,
filter_func = function(x) {
x
},
CV = NULL,
na.rm = FALSE,
...
)
model_radar_gun(
time,
velocity,
weights = 1,
CV = NULL,
use_observed_MSS = FALSE,
na.rm = FALSE,
...
)
model_laser_gun(
time,
velocity,
weights = 1,
CV = NULL,
use_observed_MSS = FALSE,
na.rm = FALSE,
...
)
model_tether(
distance,
velocity,
weights = 1,
CV = NULL,
use_observed_MSS = FALSE,
na.rm = FALSE,
...
)
model_tether_DC(
distance,
velocity,
weights = 1,
CV = NULL,
use_observed_MSS = FALSE,
na.rm = FALSE,
...
)
model_time_distance(time, distance, weights = 1, CV = NULL, na.rm = FALSE, ...)
model_time_distance_FD(
time,
distance,
weights = 1,
CV = NULL,
na.rm = FALSE,
...
)
model_time_distance_FD_fixed(
time,
distance,
weights = 1,
FD = 0,
CV = NULL,
na.rm = FALSE,
...
)
model_time_distance_TC(
time,
distance,
weights = 1,
CV = NULL,
na.rm = FALSE,
...
)
model_time_distance_DC(
time,
distance,
weights = 1,
CV = NULL,
na.rm = FALSE,
...
)
model_time_distance_TC_DC(
time,
distance,
weights = 1,
CV = NULL,
na.rm = FALSE,
...
)
model_timing_gates(distance, time, weights = 1, CV = NULL, na.rm = FALSE, ...)
model_timing_gates_TC(
distance,
time,
weights = 1,
CV = NULL,
na.rm = FALSE,
...
)
model_timing_gates_FD(
distance,
time,
weights = 1,
CV = NULL,
na.rm = FALSE,
...
)
model_timing_gates_FD_fixed(
distance,
time,
weights = 1,
FD = 0,
CV = NULL,
na.rm = FALSE,
...
)
model_timing_gates_DC(
distance,
time,
weights = 1,
CV = NULL,
na.rm = FALSE,
...
)
model_timing_gates_TC_DC(
distance,
time,
weights = 1,
CV = NULL,
na.rm = FALSE,
...
)
Arguments
- weights
Numeric vector. Default is 1
- acceleration_threshold
Acceleration cutoff. Default is 0
- velocity_threshold
Velocity cutoff. If
NULL
(default), velocity of the observation with the fastest acceleration is taken as the cutoff value (after applying thefilter_func
)- velocity_step
Velocity increment size for finding max acceleration. Default is 0.2 m/s
- n_observations
Number of top acceleration observations to keep in velocity bracket. Default is 2
- filter_func
Function to filter outliers within each velocity bracket. This is used to remove noise in the data. Default is
function(x){x}
which doesn't involve any filtering.- CV
Should cross-validation be used to estimate model fit? Default is
NULL
. Otherwise use integer indicating number of folds- na.rm
Logical. Default is FALSE
- ...
Forwarded to
nlsLM
function- time, velocity, distance, acceleration
Numeric vector
- use_observed_MSS
Should observed peak
velocity
be used asMSS
parameter? Default isFALSE
- FD
Flying distance parameter. Default is 0
Value
List object with the following elements:
- data
Data frame used to estimate the sprint parameters
- model_info
Extra information regarding model used
- model
Model returned by the
nlsLM
function- parameters
List with the following estimated parameters:
MSS
,MAC
,TAU
, andPMAX
- correction
List with additional model correcitons
- predictions
Data frame with
.predictor
,.observed
,.predicted
, and.residual
columns- model_fit
List with multiple model fit estimators
- CV
If cross-validation is performed, this will included the data as above, but for each fold
References
Samozino P. 2018. A Simple Method for Measuring Force, Velocity and Power Capabilities and Mechanical Effectiveness During Sprint Running. In: Morin J-B, Samozino P eds. Biomechanics of Training and Testing. Cham: Springer International Publishing, 237–267. DOI: 10.1007/978-3-319-05633-3_11.
Clavel, P., Leduc, C., Morin, J.-B., Buchheit, M., & Lacome, M. (2023). Reliability of individual acceleration-speed profile in-situ in elite youth soccer players. Journal of Biomechanics, 153, 111602. https://doi.org/10.1016/j.jbiomech.2023.111602
Morin, J.-B. (2021). The “in-situ” acceleration-speed profile for team sports: testing players without testing them. JB Morin, PhD – Sport Science website. Accessed 31. Dec. 2023. https://jbmorin.net/2021/07/29/the-in-situ-sprint-profile-for-team-sports-testing-players-without-testing-them/
Examples
# Model In-Situ (Embedded profiling)
data("LPS_session")
m1 <- model_in_situ(
velocity = LPS_session$velocity,
acceleration = LPS_session$acceleration,
# Use specific cutoff value
velocity_threshold = 4)
m1
#> Estimated model parameters
#> --------------------------
#> MSS MAC TAU PMAX
#> 8.175298 7.892268 1.035862 16.130411
#>
#> Estimated model corrections
#> --------------------------
#> velocity_threshold velocity_step n_observations
#> 4.0 0.2 2.0
#>
#> Model fit estimators
#> --------------------
#> R2 meanErr meanErr_perc minErr minErr_perc
#> 9.621382e-01 4.333708e-11 -2.172739e+00 -2.850020e-01 -3.774861e+01
#> maxErr maxErr_perc maxAbsErr maxAbsErr_perc RMSE
#> 2.876032e-01 9.416731e+00 2.876032e-01 3.774861e+01 1.732541e-01
#> RMSE_perc MAE MAE_perc
#> 1.192616e+01 1.507568e-01 8.054781e+00
plot(m1)
# Model Radar Gun (includes Time Correction)
df <- create_sprint_trace(MSS = 8, MAC = 6, time = seq(0, 6, 0.1))
# Add some noise
df$velocity <- df$velocity + rnorm(n = nrow(df), 0, 10^-2)
m1 <- model_radar_gun(time = df$time, velocity = df$velocity)
m1
#> Estimated model parameters
#> --------------------------
#> MSS MAC TAU PMAX
#> 8.001248 6.001633 1.333178 12.005138
#>
#> Estimated model corrections
#> --------------------------
#> TC
#> 0.001152242
#>
#> Model fit estimators
#> --------------------
#> R2 meanErr meanErr_perc minErr minErr_perc
#> 9.999738e-01 -4.845986e-09 8.423650e-01 -2.392493e-02 -1.716745e+00
#> maxErr maxErr_perc maxAbsErr maxAbsErr_perc RMSE
#> 2.647852e-02 5.058495e+01 2.647852e-02 5.058495e+01 1.063029e-02
#> RMSE_perc MAE MAE_perc
#> 6.486331e+00 7.840910e-03 1.012165e+00
plot(m1)
# Model Laser Gun (includes Time Correction)
df <- create_sprint_trace(MSS = 8, MAC = 6, time = seq(0, 6, 0.1))
# Add some noise
df$velocity <- df$velocity + rnorm(n = nrow(df), 0, 10^-2)
m1 <- model_laser_gun(time = df$time, velocity = df$velocity)
m1
#> Estimated model parameters
#> --------------------------
#> MSS MAC TAU PMAX
#> 7.999801 6.008450 1.331425 12.016601
#>
#> Estimated model corrections
#> --------------------------
#> TC
#> 0.0007096585
#>
#> Model fit estimators
#> --------------------
#> R2 meanErr meanErr_perc minErr minErr_perc
#> 9.999774e-01 -2.784258e-09 6.134959e-01 -2.859492e-02 -6.493664e-01
#> maxErr maxErr_perc maxAbsErr maxAbsErr_perc RMSE
#> 2.073111e-02 3.587191e+01 2.859492e-02 3.587191e+01 9.869609e-03
#> RMSE_perc MAE MAE_perc
#> 4.607405e+00 7.827059e-03 7.619518e-01
plot(m1)
# Model Tether
df <- create_sprint_trace(MSS = 8, MAC = 6, time = seq(0, 6, 0.5))
m1 <- model_tether(distance = df$distance, velocity = df$velocity)
m1
#> Estimated model parameters
#> --------------------------
#> MSS MAC TAU PMAX
#> 8.000000 6.000000 1.333333 12.000000
#>
#> Model fit estimators
#> --------------------
#> R2 meanErr meanErr_perc minErr minErr_perc
#> 1.000000e+00 2.732857e-16 NaN -8.881784e-16 NaN
#> maxErr maxErr_perc maxAbsErr maxAbsErr_perc RMSE
#> 8.881784e-16 NaN 8.881784e-16 NaN 6.033984e-16
#> RMSE_perc MAE MAE_perc
#> NaN 4.099285e-16 NaN
plot(m1)
# Model Tether with Distance Correction (DC)
df <- create_sprint_trace(MSS = 8, MAC = 6, time = seq(0.001, 6, 0.5), DC = 5)
m1 <- model_tether_DC(distance = df$distance, velocity = df$velocity)
m1
#> Estimated model parameters
#> --------------------------
#> MSS MAC TAU PMAX
#> 8.000000 6.000000 1.333333 12.000000
#>
#> Estimated model corrections
#> --------------------------
#> DC
#> 5
#>
#> Model fit estimators
#> --------------------
#> R2 meanErr meanErr_perc minErr minErr_perc
#> 1.000000e+00 6.524606e-09 1.087130e-04 -2.443290e-11 -3.930459e-10
#> maxErr maxErr_perc maxAbsErr maxAbsErr_perc RMSE
#> 7.824384e-08 1.304553e-03 7.824384e-08 1.304553e-03 2.258707e-08
#> RMSE_perc MAE MAE_perc
#> 3.765920e-04 6.539070e-09 1.087132e-04
plot(m1)
# Model Time-Distance trace (simple, without corrections)
df <- create_sprint_trace(MSS = 8, MAC = 6, time = seq(0, 5, by = 0.5))
m1 <- model_time_distance(time = df$time, distance = df$distance)
m1
#> Estimated model parameters
#> --------------------------
#> MSS MAC TAU PMAX
#> 8.000000 6.000000 1.333333 12.000000
#>
#> Model fit estimators
#> --------------------
#> R2 meanErr meanErr_perc minErr minErr_perc
#> 1.000000e+00 2.906766e-15 NaN 0.000000e+00 NaN
#> maxErr maxErr_perc maxAbsErr maxAbsErr_perc RMSE
#> 7.105427e-15 NaN 7.105427e-15 NaN 3.787206e-15
#> RMSE_perc MAE MAE_perc
#> NaN 2.906766e-15 NaN
plot(m1)
# Model Time-Distance trace (with Flying Distance Correction)
df <- create_sprint_trace(MSS = 8, MAC = 6, time = seq(0, 5, by = 0.5), FD = 0.5)
m1 <- model_time_distance_FD(time = df$time, distance = df$distance)
m1
#> Estimated model parameters
#> --------------------------
#> MSS MAC TAU PMAX
#> 8.000000 6.000000 1.333333 12.000000
#>
#> Estimated model corrections
#> --------------------------
#> FD
#> 0.5
#>
#> Model fit estimators
#> --------------------
#> R2 meanErr meanErr_perc minErr minErr_perc
#> 1.000000e+00 4.339963e-16 -Inf -3.108624e-15 -Inf
#> maxErr maxErr_perc maxAbsErr maxAbsErr_perc RMSE
#> 7.105427e-15 4.157878e-14 7.105427e-15 Inf 2.639395e-15
#> RMSE_perc MAE MAE_perc
#> Inf 1.503848e-15 Inf
plot(m1)
# Model Time-Distance trace (with Flying Distance Correction fixed)
df <- create_sprint_trace(MSS = 8, MAC = 6, time = seq(0, 5, by = 0.5), FD = 0.5)
m1 <- model_time_distance_FD_fixed(time = df$time, distance = df$distance, FD = 0.5)
m1
#> Estimated model parameters
#> --------------------------
#> MSS MAC TAU PMAX
#> 8.000000 6.000000 1.333333 12.000000
#>
#> Estimated model corrections
#> --------------------------
#> FD
#> 0.5
#>
#> Model fit estimators
#> --------------------
#> R2 meanErr meanErr_perc minErr minErr_perc
#> 1.000000e+00 1.453383e-15 NaN -1.776357e-15 NaN
#> maxErr maxErr_perc maxAbsErr maxAbsErr_perc RMSE
#> 3.552714e-15 NaN 3.552714e-15 NaN 2.454390e-15
#> RMSE_perc MAE MAE_perc
#> NaN 2.099331e-15 NaN
plot(m1)
# Model Time-Distance trace (with Time Correction)
df <- create_sprint_trace(MSS = 8, MAC = 6, time = seq(0, 5, by = 0.5), TC = 1.5)
m1 <- model_time_distance_TC(time = df$time, distance = df$distance)
m1
#> Estimated model parameters
#> --------------------------
#> MSS MAC TAU PMAX
#> 1895.253146 2.147529 882.527350 1017.527810
#>
#> Estimated model corrections
#> --------------------------
#> TC
#> 0.8584909
#>
#> Model fit estimators
#> --------------------
#> R2 meanErr meanErr_perc minErr minErr_perc
#> 0.9972266 -0.2001508 -Inf -0.7916282 -Inf
#> maxErr maxErr_perc maxAbsErr maxAbsErr_perc RMSE
#> 0.3846147 3.5063408 0.7916282 Inf 0.4266725
#> RMSE_perc MAE MAE_perc
#> Inf 0.3549891 Inf
plot(m1)
# Model Time-Distance trace (with Distance Correction)
df <- create_sprint_trace(MSS = 8, MAC = 6, time = seq(0, 5, by = 0.5), DC = -5)
m1 <- model_time_distance_DC(time = df$time, distance = df$distance)
m1
#> Estimated model parameters
#> --------------------------
#> MSS MAC TAU PMAX
#> 8.000000 6.000000 1.333333 12.000000
#>
#> Estimated model corrections
#> --------------------------
#> DC
#> -5
#>
#> Model fit estimators
#> --------------------
#> R2 meanErr meanErr_perc minErr minErr_perc
#> 1.000000e+00 -6.459479e-16 -1.956718e-14 -7.105427e-15 -1.351823e-13
#> maxErr maxErr_perc maxAbsErr maxAbsErr_perc RMSE
#> 3.552714e-15 6.546637e-14 7.105427e-15 1.351823e-13 2.834085e-15
#> RMSE_perc MAE MAE_perc
#> 4.958677e-14 1.937844e-15 3.147015e-14
plot(m1)
# Model Time-Distance trace (with Time and Distance Corrections)
df <- create_sprint_trace(MSS = 8, MAC = 6, time = seq(0, 5, by = 0.5), TC = -1.3, DC = 5)
m1 <- model_time_distance_TC_DC(time = df$time, distance = df$distance)
m1
#> Estimated model parameters
#> --------------------------
#> MSS MAC TAU PMAX
#> 8.000000 6.000000 1.333333 12.000000
#>
#> Estimated model corrections
#> --------------------------
#> TC DC
#> -1.3 5.0
#>
#> Model fit estimators
#> --------------------
#> R2 meanErr meanErr_perc minErr minErr_perc
#> 1.000000e+00 2.099331e-15 9.163848e-15 0.000000e+00 0.000000e+00
#> maxErr maxErr_perc maxAbsErr maxAbsErr_perc RMSE
#> 7.105427e-15 2.028565e-14 7.105427e-15 2.028565e-14 3.344769e-15
#> RMSE_perc MAE MAE_perc
#> 1.257285e-14 2.099331e-15 9.163848e-15
plot(m1)
# Model Timing Gates (simple, without corrections)
df <- create_sprint_trace(MSS = 8, MAC = 6, distance = c(5, 10, 20, 30, 40))
m1 <- model_timing_gates(distance = df$distance, time = df$time)
m1
#> Estimated model parameters
#> --------------------------
#> MSS MAC TAU PMAX
#> 8.000000 6.000000 1.333333 12.000000
#>
#> Model fit estimators
#> --------------------
#> R2 meanErr meanErr_perc minErr minErr_perc
#> 1.000000e+00 4.884981e-16 1.298446e-14 0.000000e+00 0.000000e+00
#> maxErr maxErr_perc maxAbsErr maxAbsErr_perc RMSE
#> 8.881784e-16 1.885372e-14 8.881784e-16 1.885372e-14 6.040266e-16
#> RMSE_perc MAE MAE_perc
#> 1.463129e-14 4.884981e-16 1.298446e-14
plot(m1)
# Model Timing Gates (with Time Correction)
df <- create_sprint_trace(MSS = 8, MAC = 6, distance = c(5, 10, 20, 30, 40), TC = 0.2)
m1 <- model_timing_gates_TC(distance = df$distance, time = df$time)
m1
#> Estimated model parameters
#> --------------------------
#> MSS MAC TAU PMAX
#> 8.000000 6.000000 1.333333 12.000000
#>
#> Estimated model corrections
#> --------------------------
#> TC
#> 0.2
#>
#> Model fit estimators
#> --------------------
#> R2 meanErr meanErr_perc minErr minErr_perc
#> 1.000000e+00 -3.108624e-16 -5.185586e-15 -8.881784e-16 -1.690737e-14
#> maxErr maxErr_perc maxAbsErr maxAbsErr_perc RMSE
#> 4.440892e-16 1.737815e-14 8.881784e-16 1.737815e-14 6.040266e-16
#> RMSE_perc MAE MAE_perc
#> 1.368691e-14 4.884981e-16 1.213685e-14
plot(m1)
# Model Timing Gates (with Flying Distance Correction)
df <- create_sprint_trace(MSS = 8, MAC = 6, distance = c(5, 10, 20, 30, 40), FD = 0.5)
m1 <- model_timing_gates_FD(distance = df$distance, time = df$time)
m1
#> Estimated model parameters
#> --------------------------
#> MSS MAC TAU PMAX
#> 8.000000 6.000000 1.333333 12.000000
#>
#> Estimated model corrections
#> --------------------------
#> FD
#> 0.5
#>
#> Model fit estimators
#> --------------------
#> R2 meanErr meanErr_perc minErr minErr_perc
#> 1.000000e+00 -6.217249e-16 -2.125254e-14 -8.881784e-16 -3.709322e-14
#> maxErr maxErr_perc maxAbsErr maxAbsErr_perc RMSE
#> -4.440892e-16 -1.310130e-14 8.881784e-16 3.709322e-14 6.586907e-16
#> RMSE_perc MAE MAE_perc
#> 2.290012e-14 6.217249e-16 2.125254e-14
plot(m1)
# Model Timing Gates (with Flying Distance Correction fixed)
df <- create_sprint_trace(MSS = 8, MAC = 6, distance = c(5, 10, 20, 30, 40), FD = 0.5)
m1 <- model_timing_gates_FD_fixed(distance = df$distance, time = df$time)
m1
#> Estimated model parameters
#> --------------------------
#> MSS MAC TAU PMAX
#> 7.6084315 10.4790776 0.7260593 19.9323362
#>
#> Estimated model corrections
#> --------------------------
#> FD
#> 0
#>
#> Model fit estimators
#> --------------------
#> R2 meanErr meanErr_perc minErr minErr_perc
#> 0.999604814 -0.003592961 -0.653207665 -0.056941052 -4.756087605
#> maxErr maxErr_perc maxAbsErr maxAbsErr_perc RMSE
#> 0.042158194 1.243729935 0.056941052 4.756087605 0.035338917
#> RMSE_perc MAE MAE_perc
#> 2.221121704 0.030642700 1.441672645
plot(m1)
# Model Timing Gates (with Distance Correction)
df <- create_sprint_trace(MSS = 8, MAC = 6, distance = c(5, 10, 20, 30, 40), DC = 1.5)
m1 <- model_timing_gates_DC(distance = df$distance, time = df$time)
m1
#> Estimated model parameters
#> --------------------------
#> MSS MAC TAU PMAX
#> 8.000000 6.000000 1.333333 12.000000
#>
#> Estimated model corrections
#> --------------------------
#> DC
#> 1.5
#>
#> Model fit estimators
#> --------------------
#> R2 meanErr meanErr_perc minErr minErr_perc
#> 1.000000e+00 1.332268e-16 -6.621710e-16 -2.220446e-16 -1.779418e-14
#> maxErr maxErr_perc maxAbsErr maxAbsErr_perc RMSE
#> 8.881784e-16 1.448332e-14 8.881784e-16 1.779418e-14 4.094300e-16
#> RMSE_perc MAE MAE_perc
#> 1.026060e-14 2.220446e-16 6.455500e-15
plot(m1)
# Model Timing Gates (with Time and Distance Corrections)
df <- create_sprint_trace(MSS = 8, MAC = 6, distance = c(5, 10, 20, 30, 40), TC = 0.25, DC = 1.5)
m1 <- model_timing_gates_TC_DC(distance = df$distance, time = df$time)
m1
#> Estimated model parameters
#> --------------------------
#> MSS MAC TAU PMAX
#> 8.000000 6.000000 1.333333 12.000000
#>
#> Estimated model corrections
#> --------------------------
#> TC DC
#> 0.25 1.50
#>
#> Model fit estimators
#> --------------------
#> R2 meanErr meanErr_perc minErr minErr_perc
#> 1.000000e+00 2.220446e-16 7.152383e-15 -4.440892e-16 -1.167730e-14
#> maxErr maxErr_perc maxAbsErr maxAbsErr_perc RMSE
#> 8.881784e-16 1.869898e-14 8.881784e-16 1.869898e-14 4.965068e-16
#> RMSE_perc MAE MAE_perc
#> 1.341212e-14 3.996803e-16 1.182330e-14
plot(m1)